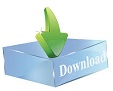
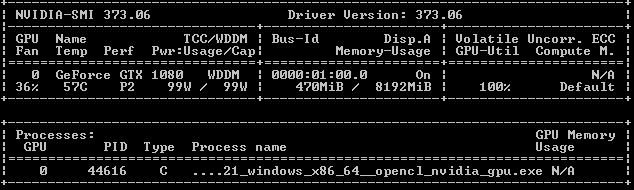
We demonstrate the fault-tolerance of the platform in self-recovering from unexpected disconnections of volunteer devices. We show that our platform adapts well to the changing environment getting a numerical accuracy similar to today’s configurations using a given number of homogeneous (hardware and software) computers as a static platform for learning. The public open-source platform enables interoperability between volunteers connected using web browsers and Python processes. Thus, we conduct comprehensive empirical analysis in a static configuration and highly dynamic and changing scenarios. The platform dynamically self-adapts to variations in the number of collaborating heterogeneous devices due to unexpected disconnections (i.e., volunteers can join and leave at any time). The proposed decentralized and adaptive system architecture for asynchronous learning allows volunteer users to yield their device resources and local data to train a shared ML model. We then evaluate its results on problems relevant to FEEL.
#Formula boinc software
We propose, implement, and evaluate a new software platform for this purpose. Our research focuses on adapting the learning when the number of volunteers is low and may even drop to zero. In this work, we propose an algorithm for FEEL that adapts to asynchronous clients joining and leaving the computation. The combination of both is known as federated edge learning (FEEL). On the other hand, federated learning (FL) deals with training a shared machine learning (ML) model in a distributed (non-centralized) manner while keeping private data locally on edge devices. On the one hand, edge computing (EC) suggests to increase processing on edge devices.

Recently, two related paradigms try to address the above problems. However, studying these data and gaining insights from them is still of great relevance to science and society. These devices produce a large amount of data that cannot be analyzed in any data center or stored in the cloud, and it might be private or sensitive, thus precluding existing classic approaches. The number of devices, from smartphones to IoT hardware, interconnected via the Internet is growing all the time. Our evaluation results demonstrate that the proposed prediction schemes outperform well-known common approaches in terms of accuracy, and actually can help users in a shared resource environment to run their large-scale applications by effectively forecasting various computing resource capacity and performance. We performed quantitative analysis and conducted microbenchmark experiments on a real multinational shared computing platform. This paper presents effective resource profiling and performance prediction models including Adaptive Filter-based Online Linear Regression (AFOLR) and Adaptive Filter-based Moving Average (AFMV) based on the linear difference equation combining past predicted values and recent profiled information, which aim to support large-scale applications in distributed scientific computing environments. An effective VO’s resource profiling and modeling system can address these problems by forecasting resource characteristics and availability. Furthermore, shared resources are inconsistent, making it difficult to accurately forecast resource capacity. However, VOs often suffer from providing exact dynamic resource information due to their scale and autonomous resource management policies. Over the past decade, VOs have been proven to be a powerful research testbed for accessing massive amount of computing resources shared by several organizations at almost no cost. As a result, many institutions collaborate to solve large-scale problems by creating virtual organizations (VOs), and integrate hundreds of thousands of geographically distributed heterogeneous computing resources. Our primary goal for the 2021 season will be to get back up into League 1.Scientific applications often require substantial amount of computing resources for running challenging jobs potentially consisting of many tasks from hundreds of thousands to even millions. The OCN team was relegated to League 2 for the 2021 season.
#Formula boinc full
See the Formula BOINC home page for a full breakdown of the rules: Formula Boinc Points are amassed based on a team's individual project ranking throughout the year and by their ranking in each of the 23 sprints. Poll is up top, so let me know if you guys want to make a run at getting back into League 1.įormula BOINC is a year long BOINC team competition based on the structure of the Formula 1 Championship. We were pretty active in Formula BOINC in the past, but with the overall slow-down of activity since the site migration, we were basically a non-entity in the competition last year. I am looking to gauge the interest of the team in the Formula BOINC competition.
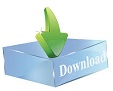